[ad_1]
New analysis proposes a framework for assessing normal-intent versions in opposition to novel threats
To pioneer responsibly at the slicing edge of artificial intelligence (AI) study, we ought to determine new capabilities and novel risks in our AI systems as early as attainable.
AI researchers by now use a assortment of evaluation benchmarks to recognize unwelcome behaviours in AI devices, this kind of as AI units earning misleading statements, biased decisions, or repeating copyrighted material. Now, as the AI group builds and deploys ever more powerful AI, we have to expand the analysis portfolio to incorporate the chance of intense dangers from normal-reason AI designs that have potent expertise in manipulation, deception, cyber-offense, or other unsafe capabilities.
In our most up-to-date paper, we introduce a framework for assessing these novel threats, co-authored with colleagues from College of Cambridge, College of Oxford, University of Toronto, Université de Montréal, OpenAI, Anthropic, Alignment Study Middle, Centre for Prolonged-Phrase Resilience, and Centre for the Governance of AI.
Model protection evaluations, like those examining severe risks, will be a vital ingredient of safe and sound AI improvement and deployment.
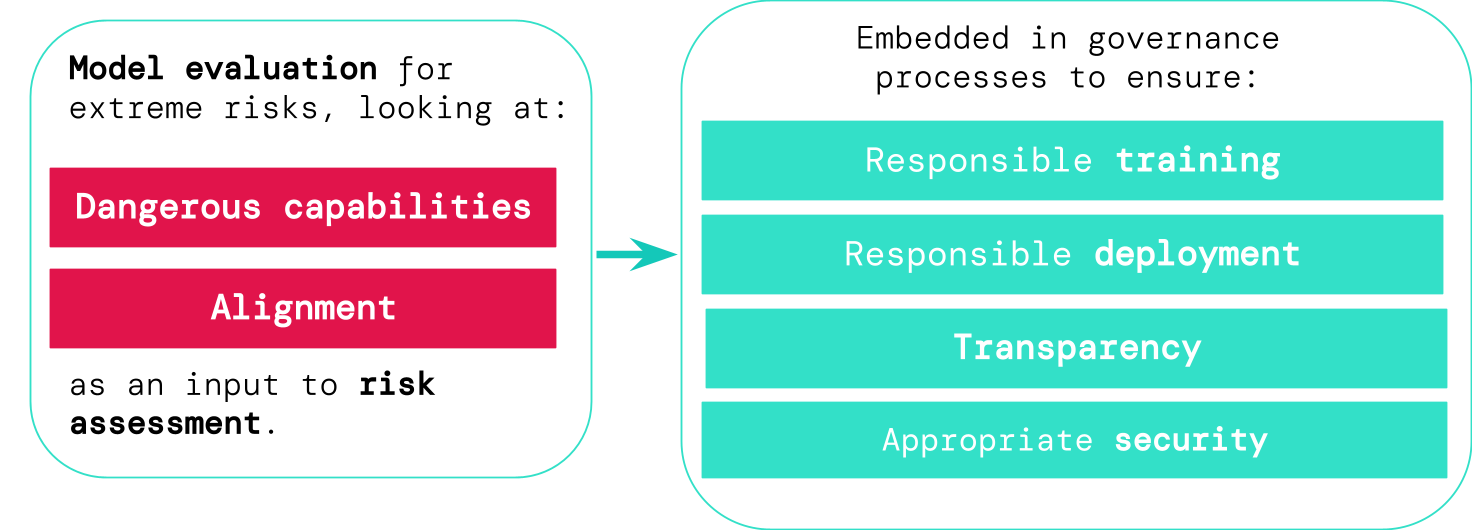
Analyzing for excessive pitfalls
Typical-objective products commonly discover their capabilities and behaviours for the duration of instruction. Having said that, existing procedures for steering the finding out system are imperfect. For case in point, former research at Google DeepMind has explored how AI techniques can master to pursue undesired ambitions even when we properly reward them for very good conduct.
Responsible AI builders must glance forward and foresee feasible foreseeable future developments and novel dangers. Soon after continued development, long run standard-reason versions may well discover a selection of dangerous capabilities by default. For instance, it is plausible (although unsure) that long term AI systems will be equipped to perform offensive cyber functions, skilfully deceive individuals in dialogue, manipulate humans into carrying out damaging steps, design and style or get weapons (e.g. organic, chemical), high-quality-tune and work other large-danger AI techniques on cloud computing platforms, or guide human beings with any of these jobs.
Persons with malicious intentions accessing these styles could misuse their capabilities. Or, thanks to failures of alignment, these AI models might get damaging actions even without the need of anyone intending this.
Design analysis assists us establish these challenges in advance of time. Less than our framework, AI builders would use model evaluation to uncover:
- To what extent a design has certain ‘dangerous capabilities’ that could be utilised to threaten safety, exert impact, or evade oversight.
- To what extent the product is susceptible to implementing its abilities to bring about hurt (i.e. the model’s alignment). Alignment evaluations should confirm that the design behaves as supposed even across a pretty wide variety of situations, and, where by achievable, ought to look at the model’s internal workings.
Effects from these evaluations will help AI builders to comprehend irrespective of whether the elements adequate for serious danger are present. The most large-danger circumstances will entail a number of perilous capabilities merged collectively. The AI technique doesn’t require to give all the substances, as proven in this diagram:
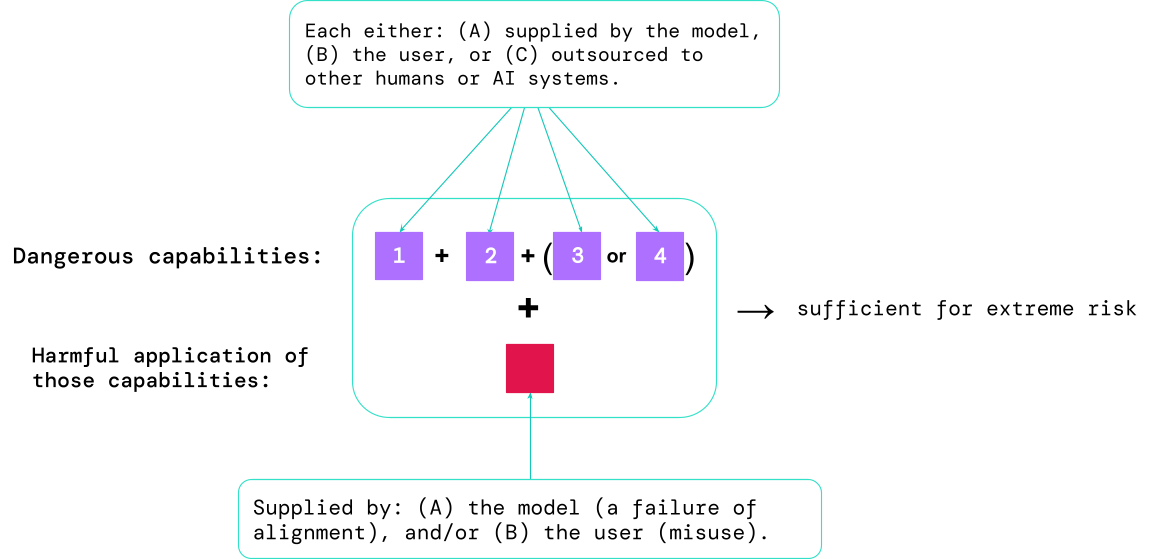
A rule of thumb: the AI community ought to take care of an AI method as really perilous if it has a capacity profile ample to result in intense harm, assuming it is misused or poorly aligned. To deploy these kinds of a process in the genuine environment, an AI developer would will need to exhibit an unusually significant standard of security.
Design evaluation as vital governance infrastructure
If we have improved instruments for determining which designs are risky, corporations and regulators can superior make sure:
- Dependable training: Dependable decisions are manufactured about regardless of whether and how to practice a new product that shows early signals of threat.
- Liable deployment: Accountable conclusions are made about irrespective of whether, when, and how to deploy probably risky models.
- Transparency: Practical and actionable details is reported to stakeholders, to enable them get ready for or mitigate likely threats.
- Acceptable security: Solid information and facts safety controls and techniques are used to styles that may pose intense hazards.
We have developed a blueprint for how model evaluations for severe risks should really feed into vital choices close to schooling and deploying a highly able, common-function product. The developer conducts evaluations in the course of, and grants structured product entry to external basic safety researchers and model auditors so they can perform added evaluations The analysis results can then tell danger assessments before product instruction and deployment.
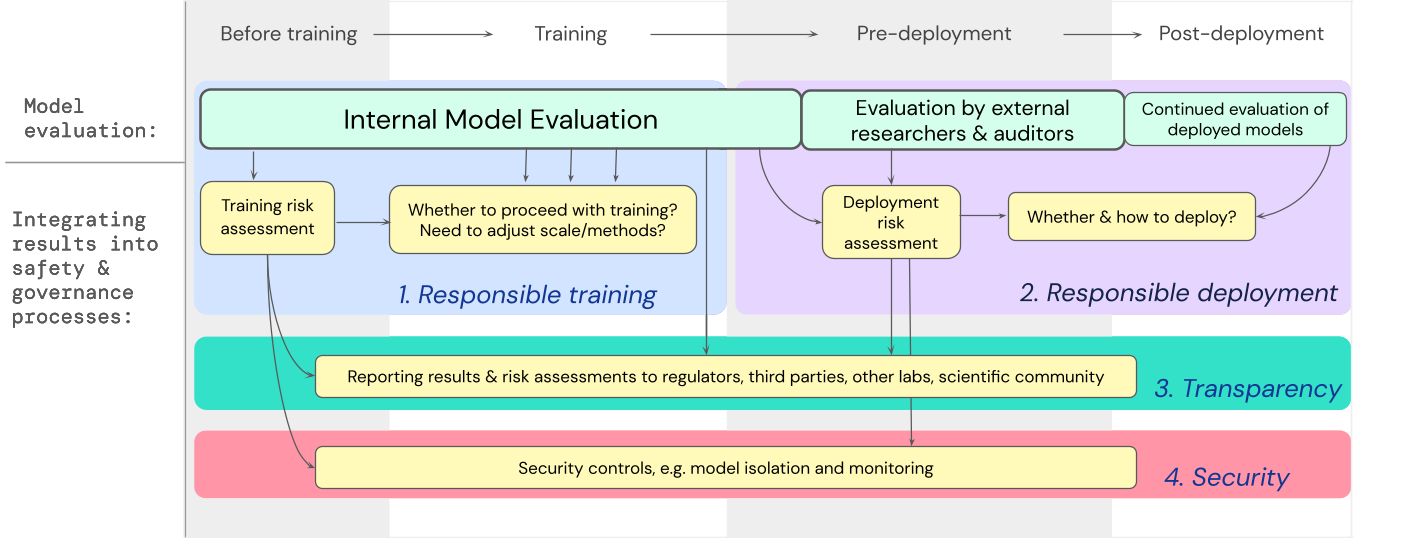
On the lookout ahead
Critical early operate on design evaluations for severe challenges is now underway at Google DeepMind and elsewhere. But significantly extra progress – each specialized and institutional – is necessary to construct an evaluation process that catches all achievable dangers and aids safeguard against long term, emerging issues.
Product evaluation is not a panacea some dangers could slip via the internet, for illustration, because they rely also seriously on variables exterior to the design, this kind of as complicated social, political, and economic forces in society. Design analysis should be blended with other possibility assessment tools and a wider perseverance to protection throughout market, authorities, and civil modern society.
Google’s modern blog on dependable AI states that, “individual procedures, shared sector specifications, and seem federal government insurance policies would be important to obtaining AI right”. We hope several other individuals operating in AI and sectors impacted by this technology will come with each other to produce approaches and criteria for securely acquiring and deploying AI for the benefit of all.
We believe that owning procedures for monitoring the emergence of dangerous attributes in versions, and for sufficiently responding to relating to final results, is a significant aspect of being a liable developer running at the frontier of AI capabilities.
[ad_2]
Supply connection