[ad_1]
Our brain has an awesome capacity to course of action visible facts. We can get one look at a advanced scene, and within just milliseconds be equipped to parse it into objects and their attributes, like color or sizing, and use this information to describe the scene in uncomplicated language. Underlying this seemingly effortless skill is a sophisticated computation carried out by our visual cortex, which includes having hundreds of thousands of neural impulses transmitted from the retina and transforming them into a additional significant variety that can be mapped to the uncomplicated language description. In get to completely fully grasp how this system performs in the mind, we need to have to determine out each how the semantically meaningful facts is represented in the firing of neurons at the end of the visible processing hierarchy, and how such a illustration may perhaps be learnt from largely untaught working experience.
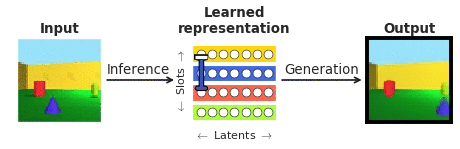
To respond to these inquiries in the context of facial area perception, we joined forces with our collaborators at Caltech (Doris Tsao) and the Chinese Academy of Science (Le Chang). We chose faces mainly because they are properly analyzed in the neuroscience community and are normally observed as a “microcosm of item recognition”. In certain, we desired to look at the responses of single cortical neurons in the face patches at the finish of the visible processing hierarchy, recorded by our collaborators to a recently emerged class of so known as “disentangling” deep neural networks that, not like the typical “black box” techniques, explicitly aim to be interpretable to individuals. A “disentangling” neural community learns to map advanced visuals into a little range of internal neurons (known as latent units), each a single representing a single semantically significant attribute of the scene, like color or size of an object (see Figure 1). As opposed to the “black box” deep classifiers qualified to recognise visible objects by way of a biologically unrealistic volume of exterior supervision, these types of disentangling products are educated devoid of an exterior teaching sign applying a self-supervised goal of reconstructing enter illustrations or photos (era in Figure 1) from their learnt latent illustration (obtained by way of inference in Figure 1).
Disentangling was hypothesised to be important in the device finding out community just about 10 several years ago as an integral part for making extra facts-productive, transferable, fair, and imaginative artificial intelligence units. However, for yrs, making a product that can disentangle in observe has eluded the subject. The very first design in a position to do this correctly and robustly, known as β-VAE, was produced by having inspiration from neuroscience: β-VAE learns by predicting its own inputs it involves equivalent visual knowledge for prosperous understanding as that encountered by infants and its learnt latent representation mirrors the qualities regarded of the visual brain.
In our new paper, we measured the extent to which the disentangled units discovered by a β-VAE skilled on a dataset of deal with illustrations or photos are very similar to the responses of solitary neurons at the end of the visible processing recorded in primates looking at the similar faces. The neural knowledge was gathered by our collaborators underneath rigorous oversight from the Caltech Institutional Animal Treatment and Use Committee. When we produced the comparison, we identified a little something stunning – it seemed like the handful of disentangled models identified by β-VAE were being behaving as if they have been equal to a likewise sized subset of the authentic neurons. When we looked closer, we discovered a sturdy one particular-to-one particular mapping in between the serious neurons and the synthetic types (see Figure 2). This mapping was considerably more robust than that for choice styles, such as the deep classifiers formerly regarded as to be state of the artwork computational designs of visual processing, or a hand-crafted design of confront notion viewed as the “gold standard” in the neuroscience community. Not only that, β-VAE units ended up encoding semantically significant information like age, gender, eye size, or the presence of a smile, enabling us to understand what characteristics one neurons in the brain use to signify faces.
.jpg)
If β-VAE was certainly able to automatically find artificial latent models that are equal to the serious neurons in terms of how they answer to face images, then it ought to be achievable to translate the activity of actual neurons into their matched artificial counterparts, and use the generator (see Determine 1) of the properly trained β-VAE to visualise what faces the actual neurons are symbolizing. To examination this, we introduced the primates with new facial area pictures that the model has hardly ever experienced, and checked if we could render them using the β-VAE generator (see Determine 3). We identified that this was indeed probable. Employing the action of as number of as 12 neurons, we were in a position to make facial area photographs that have been additional correct reconstructions of the originals and of better visible top quality than those people generated by the different deep generative styles. This is inspite of the truth that the different designs are known to be greater impression turbines than β-VAE in normal.
.jpg)
Our conclusions summarised in the new paper counsel that the visual mind can be comprehended at a single-neuron stage, even at the end of its processing hierarchy. This is contrary to the popular belief that semantically meaningful data is multiplexed among a substantial amount of these types of neurons, every single a person remaining mostly uninterpretable individually, not compared with how facts is encoded across complete levels of artificial neurons in deep classifiers. Not only that, our results advise that it is probable that the brain learns to guidance our effortless potential to do visible perception by optimising the disentanglement aim. While β-VAE was originally formulated with inspiration from high-level neuroscience rules, the utility of disentangled representations for intelligent behaviour has so much been mostly demonstrated in the machine-finding out local community. In line with the abundant historical past of mutually beneficial interactions concerning neuroscience and machine learning, we hope that the most up-to-date insights from machine learning may perhaps now feed back again to the neuroscience local community to look into the advantage of disentangled representations for supporting intelligence in organic techniques, in certain as the basis for summary reasoning, or generalisable and economical job studying.
[ad_2]
Supply backlink