[ad_1]
In new many years, major overall performance gains in autoregressive language modeling have been reached by raising the range of parameters in Transformer types. This has led to a huge increase in education electrical power expense and resulted in a generation of dense “Large Language Models” (LLMs) with 100+ billion parameters. Simultaneously, significant datasets made up of trillions of words have been gathered to facilitate the teaching of these LLMs.
We investigate an alternate route for strengthening language versions: we increase transformers with retrieval more than a database of textual content passages such as world-wide-web web pages, guides, information and code. We simply call our strategy RETRO, for “Retrieval Enhanced TRansfOrmers”.
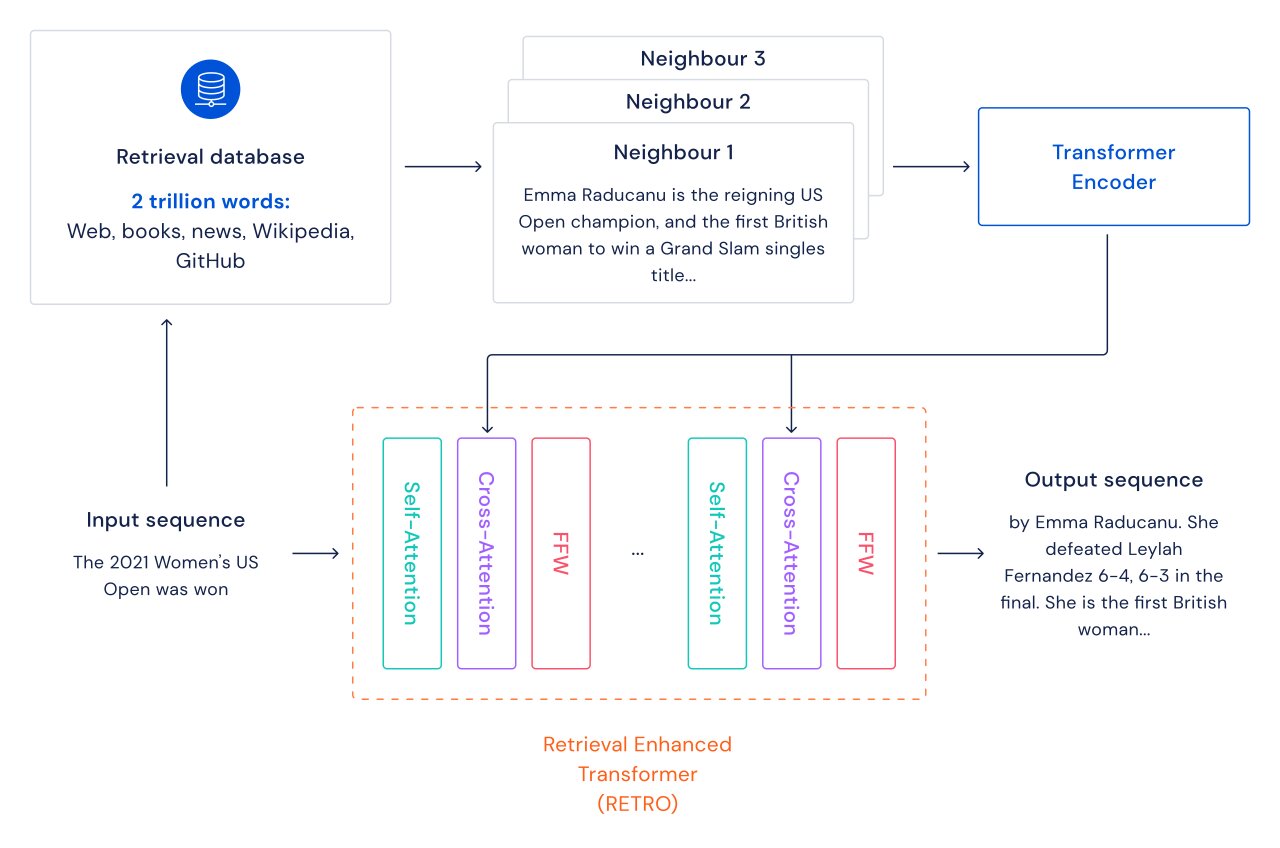
In standard transformer language types, the rewards of model size and knowledge dimension are linked: as long as the dataset is big sufficient, language modeling performance is restricted by the dimensions of the model. Nevertheless, with RETRO the product is not constrained to the data found throughout training– it has access to the total education dataset as a result of the retrieval mechanism. This final results in major functionality gains as opposed to a normal Transformer with the same quantity of parameters. We clearly show that language modeling increases repeatedly as we enhance the size of the retrieval databases, at least up to 2 trillion tokens – 175 comprehensive lifetimes of steady reading through.
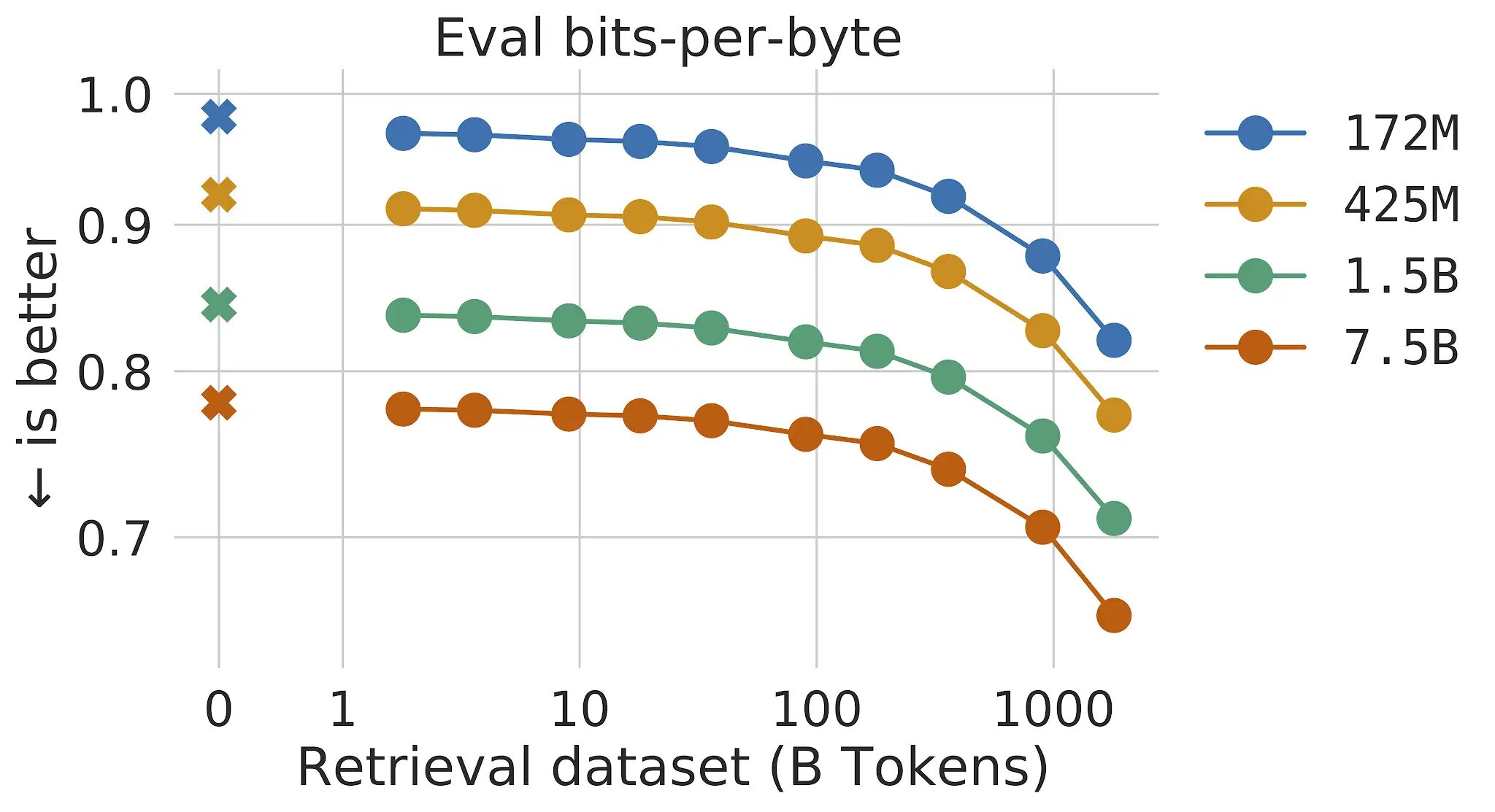
For each individual text passage (approximately a paragraph of a doc), a nearest-neighbor lookup is executed which returns equivalent sequences observed in the instruction databases, and their continuation. These sequences enable forecast the continuation of the input textual content. The RETRO architecture interleaves typical self-interest at a doc degree and cross-consideration with retrieved neighbors at a finer passage level. This benefits in each more accurate and a lot more factual continuations. Furthermore, RETRO increases the interpretability of design predictions, and offers a route for immediate interventions through the retrieval database to strengthen the safety of textual content continuation. In our experiments on the Pile, a common language modeling benchmark, a 7.5 billion parameter RETRO design outperforms the 175 billion parameter Jurassic-1 on 10 out of 16 datasets and outperforms the 280B Gopher on 9 out of 16 datasets.
Below, we clearly show two samples from our 7B baseline model and from our 7.5B RETRO model model that spotlight how RETRO’s samples are a lot more factual and keep a lot more on topic than the baseline sample.
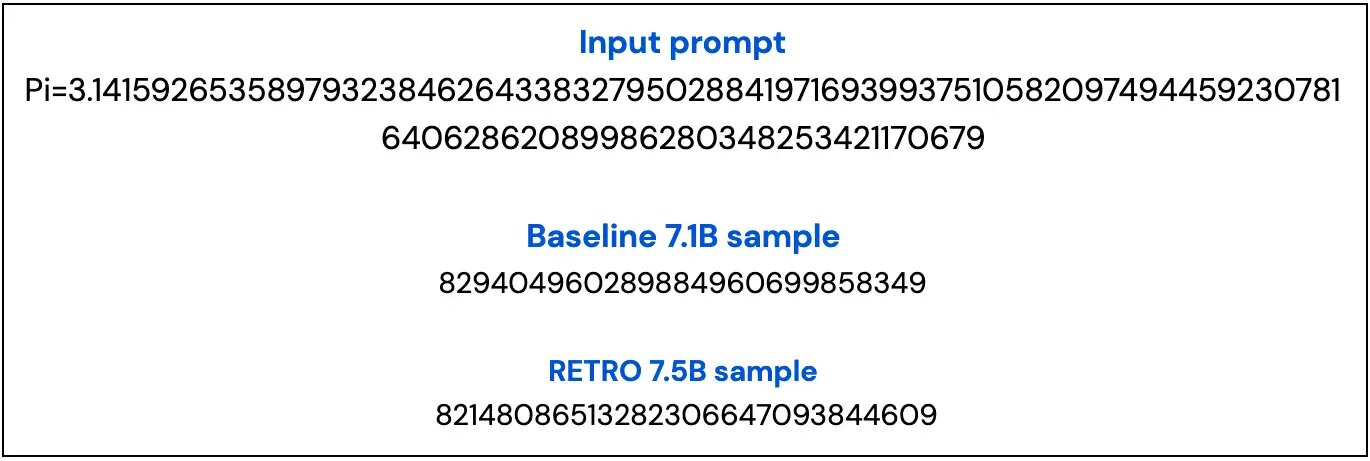
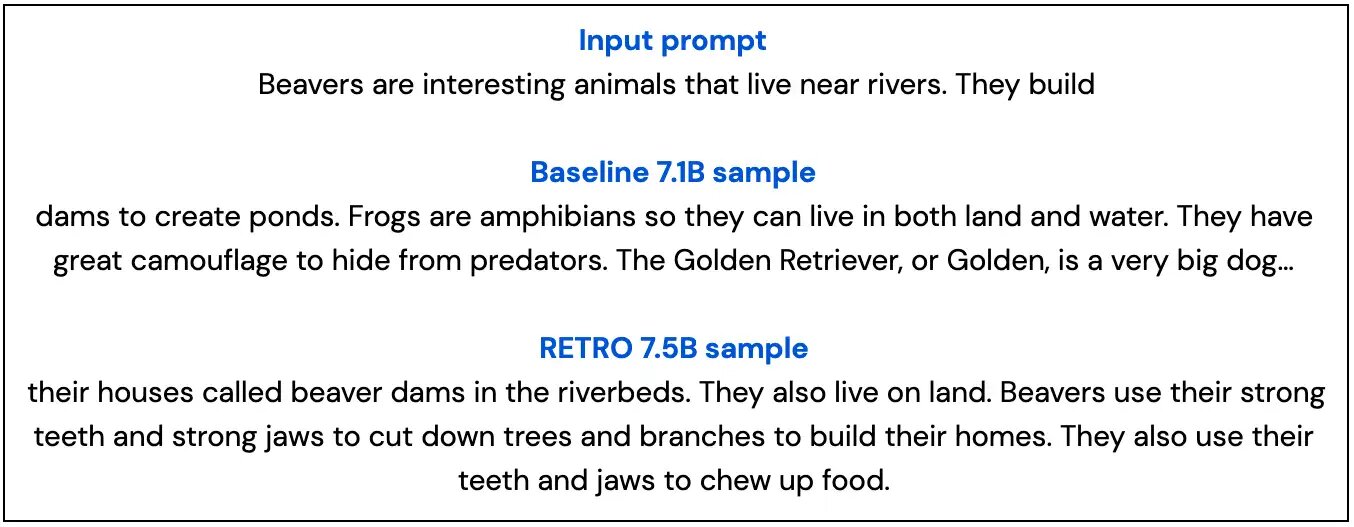
[ad_2]
Source url