[ad_1]
Engineering deployed in the true environment inevitably faces unexpected challenges. These issues arise for the reason that the natural environment where by the technological know-how was formulated differs from the atmosphere wherever it will be deployed. When a engineering transfers correctly we say it generalises. In a multi-agent program, these as autonomous car technology, there are two doable sources of generalisation trouble: (1) bodily-setting variation these types of as modifications in temperature or lighting, and (2) social-natural environment variation: adjustments in the behaviour of other interacting individuals. Handling social-natural environment variation is at least as essential as handling physical-natural environment variation, however it has been much a lot less researched.
As an example of a social environment, take into account how self-driving vehicles interact on the street with other vehicles. Just about every car or truck has an incentive to transport its very own passenger as rapidly as possible. Nonetheless, this levels of competition can guide to inadequate coordination (highway congestion) that negatively impacts everybody. If autos function cooperatively, much more passengers might get to their place a lot more quickly. This conflict is termed a social dilemma.
Nevertheless, not all interactions are social dilemmas. For occasion, there are synergistic interactions in open-resource application, there are zero-sum interactions in athletics, and coordination issues are at the main of supply chains. Navigating every single of these scenarios demands a extremely unique method.
Multi-agent reinforcement discovering provides equipment that allow us to check out how artificial agents may perhaps interact with one particular yet another and with unfamiliar people (these kinds of as human users). This class of algorithms is envisioned to conduct far better when examined for their social generalisation capabilities than many others. Nonetheless, till now, there has been no systematic analysis benchmark for evaluating this.
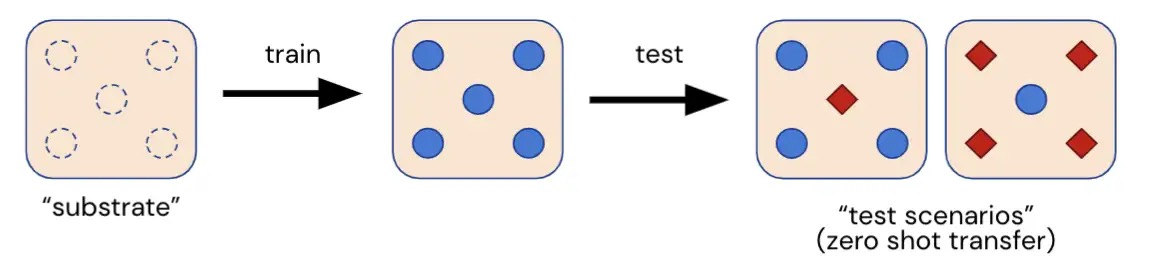
In this article we introduce Melting Pot, a scalable evaluation suite for multi-agent reinforcement finding out. Melting Pot assesses generalization to novel social conditions involving each common and unfamiliar persons, and has been intended to exam a broad vary of social interactions these as: cooperation, opposition, deception, reciprocation, have faith in, stubbornness and so on. Melting Pot delivers researchers a established of 21 MARL “substrates” (multi-agent game titles) on which to practice brokers, and in excess of 85 exceptional take a look at eventualities on which to assess these qualified brokers. The efficiency of brokers on these held-out examination eventualities quantifies irrespective of whether agents:
- Conduct properly across a variety of social situations in which people today are interdependent,
- Interact successfully with unfamiliar folks not seen during training,
- Pass a universalisation examination: answering positively to the problem “what if everyone behaved like that?”
The resulting score can then be applied to rank distinct multi-agent RL algorithms by their potential to generalise to novel social conditions.
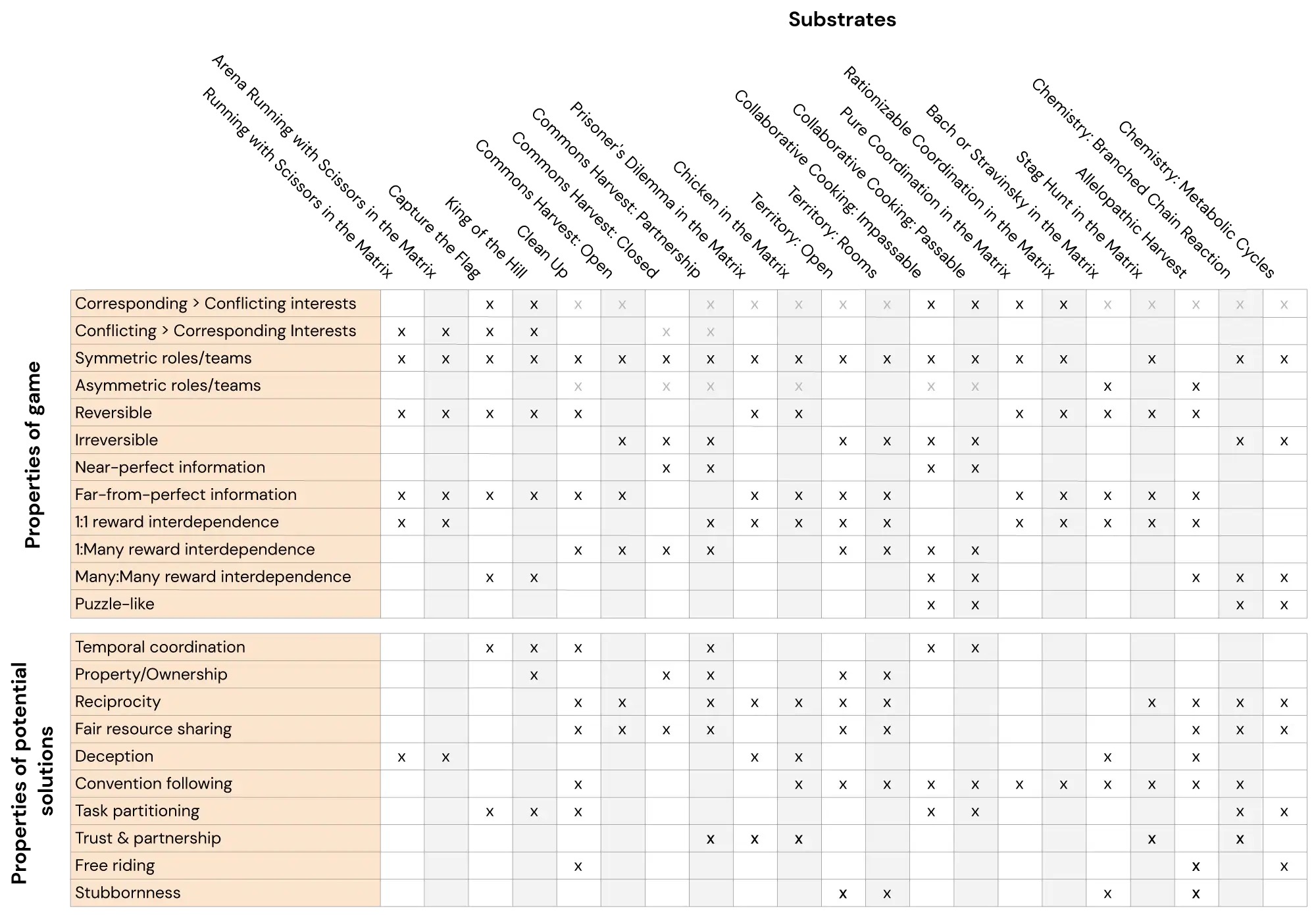
We hope Melting Pot will become a normal benchmark for multi-agent reinforcement mastering. We approach to sustain it, and will be extending it in the coming yrs to go over additional social interactions and generalisation eventualities.
Discover far more from our GitHub page.
[ad_2]
Source url